
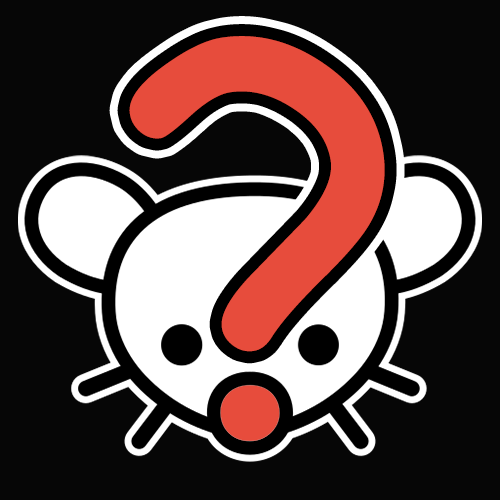
You should probably hook up with the SillyTavern crowd. It’s a frontend to chat with LLMs that will do what you want. Its main purpose is chat role-play. You can assign a persona to the LLM and ST will handle the prompt to make it work. It also handles jailbreaks if you want to use one of the big ones (no idea if it works well). You can also connect to other services that run open models, including aihorde.
https://github.com/SillyTavern/SillyTavern
https://www.reddit.com/r/SillyTavernAI/
If you want to host your own model you can find more help here:
A year as cool as the one they were born in